AI in Psychiatry:Research Finds High Accuracy in ChatGPT's Psychiatric Assessments
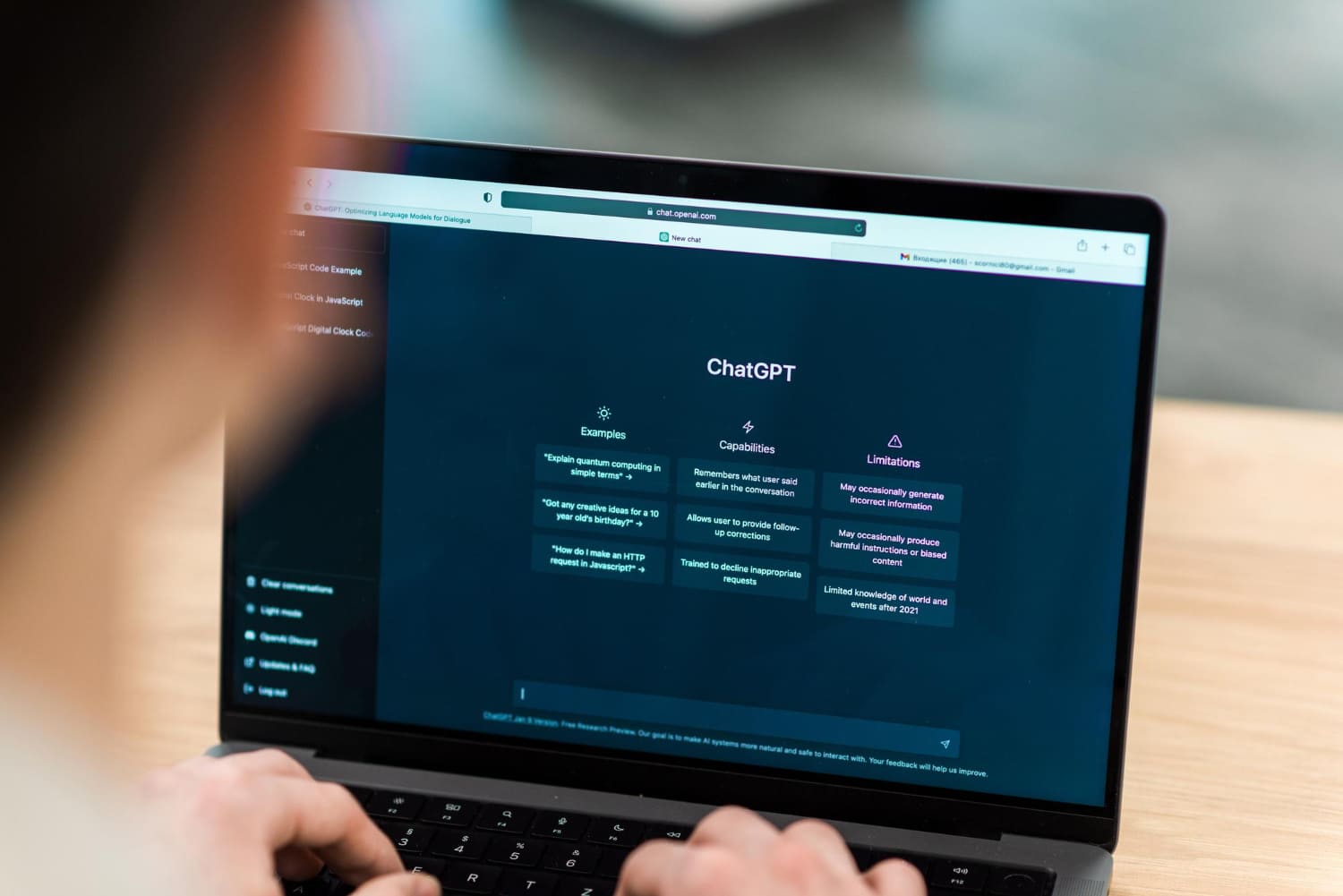
By Dr. Jash Ajmera
Artificial Intelligence (AI), particularly large language models (LLMs) like ChatGPT, is rapidly permeating various fields, including medicine. Its ability to process vast amounts of text, generate human-like responses, and assist in complex tasks has sparked interest in its potential applications in healthcare. One area receiving significant attention is psychiatry, where accurate diagnosis often relies on interpreting complex patient narratives and histories.
A notable study investigated ChatGPT's capabilities in this domain, specifically exploring how well it could perform in producing psychiatric diagnoses when presented with standardized clinical case vignettes. Research conducted by Franco D'Souza and colleagues, published in the Asian Journal of Psychiatry, provides valuable insights into this question.
The Research Question: Putting ChatGPT to the Psychiatric Test
The core objective of the study was to evaluate the performance of ChatGPT (specifically the GPT-3.5 version) on tasks central to psychiatric practice: making diagnoses, considering differential diagnoses (other possible conditions), and suggesting management strategies. Could an AI, trained on diverse internet text data, demonstrate the nuanced understanding required for these tasks?
To create a standardized and challenging test, the researchers utilized a well-established resource: the book "100 Cases in Psychiatry" by Barry Wright and colleagues. This book contains detailed clinical case vignettes, each presenting a fictional patient's history, symptoms, and relevant background information, followed by questions designed to guide diagnostic reasoning and management planning.
How Was the Study Conducted? (Methodology)
The methodology employed by D'Souza and his team was straightforward yet rigorous:
Case Presentation: Each of the 100 psychiatric case vignettes from the aforementioned book was presented as input to ChatGPT 3.5. These vignettes simulate real-world clinical encounters, providing the AI with the kind of information a psychiatrist might gather.
Response Generation: ChatGPT was prompted to respond to the information and guiding questions associated with each vignette, effectively asking it to perform a diagnostic workup and suggest management.
Evaluation: The researchers meticulously recorded ChatGPT's responses for each case.
Grading: These responses were then compared against reference answers (presumably derived from the source book or expert consensus) and graded on a scale from A to D:
A: Highest grade, indicating an excellent and accurate response.
B: Good response, largely accurate with minor omissions or points of discussion.
C: Acceptable response, but with notable shortcomings.
D: Unacceptable response, containing significant errors or being fundamentally flawed.
The evaluation likely focused on the accuracy of the primary diagnosis, the relevance of differential diagnoses considered, and the appropriateness of the proposed management strategies.
What Did the Study Find? (Key Results)
The results reported in the research were quite striking and pointed towards a significant level of competence within the AI model:
High Performance: ChatGPT achieved an 'A' grade for 61 out of the 100 vignettes and a 'B' grade for another 31 vignettes. This means that in 92% of the cases, the AI's response was considered good to excellent.
No Diagnostic Errors: Perhaps most notably, the study reported that none of ChatGPT's responses contained outright diagnostic errors or received the unacceptable 'D' grade. While 8 responses received a 'C', indicating room for improvement, the absence of critical diagnostic mistakes is significant.
Strengths: The AI performed particularly well in proposing strategies for managing the diagnosed disorders and associated symptoms. Its ability to make diagnoses and consider differential diagnoses was also strong, though slightly less proficient than its management suggestions.
Researcher Conclusion: Based on these findings, the study authors concluded, "It is evident from our study that ChatGPT 3.5 has appreciable knowledge and interpretation skills in Psychiatry." They highlighted the potential for ChatGPT to serve as a valuable AI-based tool to assist clinicians in diagnostic and treatment decisions.
Hold On, What Are the Limitations? (Crucial Considerations)
While the findings are impressive, it's essential to approach them with caution and understand the study's limitations, as well as the broader constraints of current LLM technology in sensitive fields like psychiatry. Several important caveats emerge from the research and general understanding of LLMs:
The Training Data Question: A major caveat is the uncertainty surrounding ChatGPT's training data. Was the "100 Cases in Psychiatry" book part of the vast dataset used to train GPT-3.5? ChatGPT itself cannot disclose its specific training materials. If the AI was trained on the exact questions and answers it was tested on, its high performance might be more a feat of information retrieval than genuine diagnostic reasoning. Performance could differ significantly if tested on novel case material completely unknown to it.
LLM Hallucinations and Inconsistencies: Large language models are known to sometimes "hallucinate" – generating plausible-sounding but incorrect or fabricated information. They can also produce inconsistent responses. While this specific study reported no diagnostic errors in this test set, the underlying risk remains inherent to the technology. Clinical use would require robust mechanisms to verify the AI's output.
Lack of True Understanding and Empathy: ChatGPT processes language patterns; it doesn't possess genuine understanding, consciousness, or empathy. Psychiatric diagnosis often involves interpreting subtle non-verbal cues, understanding context deeply, and building therapeutic rapport – abilities far beyond current AI. The model also struggles with tasks requiring deep personality assessment.
Version Specificity: The study utilized ChatGPT 3.5. Newer versions like GPT-4 (and beyond) might perform differently – potentially better in some areas, but perhaps with different nuances or limitations. Findings from one version don't automatically translate to others.
Need for Human Oversight and Validation: The researchers themselves, along with experts discussing AI in medicine, emphasize that tools like ChatGPT cannot replace skilled clinicians. They can potentially assist – perhaps in initial screening, information gathering, or drafting notes – but final diagnostic decisions and treatment planning must remain under the purview of trained human professionals. Reliability, validation, proper guidelines, and ethical frameworks are non-negotiable prerequisites for any AI implementation in healthcare, a point underscored in the research discussion.
Ethical and Privacy Concerns: Using AI with sensitive patient information raises significant ethical and privacy challenges that need careful consideration and robust safeguards before any clinical application.
Conclusion: Promising Potential, Proceed with Caution
The research examining ChatGPT's performance on psychiatric case vignettes offers a fascinating glimpse into the potential capabilities of LLMs in this complex field. The AI's ability to process case information and generate largely accurate diagnostic and management suggestions is noteworthy. It suggests potential future roles for AI in supporting clinicians, streamlining workflows, or even aiding in medical education.
However, the limitations identified within the study and inherent to current AI technology are substantial and critical. The ambiguity of training data, the risks of errors, the lack of genuine understanding, and the paramount importance of ethical considerations mean we are still a long way from AI psychiatrists. The findings underscore the need for cautious optimism, rigorous validation on diverse and novel data, and the development of strict safety and ethical guidelines.
ChatGPT and similar AI tools may indeed become valuable assistants in the psychiatrist's toolkit, but they are not poised to replace the human element essential to mental healthcare anytime soon. Future research must focus not only on capability but heavily on reliability, safety, and responsible integration.